by Sentimenti Team | Jan 15, 2021 | SentiBrand, Sentistocks
Cyberpunk 2077 has already premiered, and the emotions surrounding this event are extreme. The inspiration for this comparative analysis came from the comments surrounding a post made by Michał Sadowski, the creator of BRAND24, on his private Facebook profile. The entry refers to the reception of the game by Internet users. Our attention was drawn in particular to comments regarding the use by investors of sentiment as an analytical tool.
Cyberpunk 2077 and CD Projekt share price. Sentiment analysis a tool for investors?
Generally available media monitoring tools offer automatic sentiment analysis (quantitative) as standard. However, we should ask ourselves whether we can infer changes in emotions on the basis of such analysis? If the opinion was negative, how negative? Is it possible to draw conclusions about measurable parameters on the basis of such an analysis? Such a parameter is undoubtedly the price (course) of shares. But based on such a chart, was it possible to predict what would happen to the share price of CD Projekt?
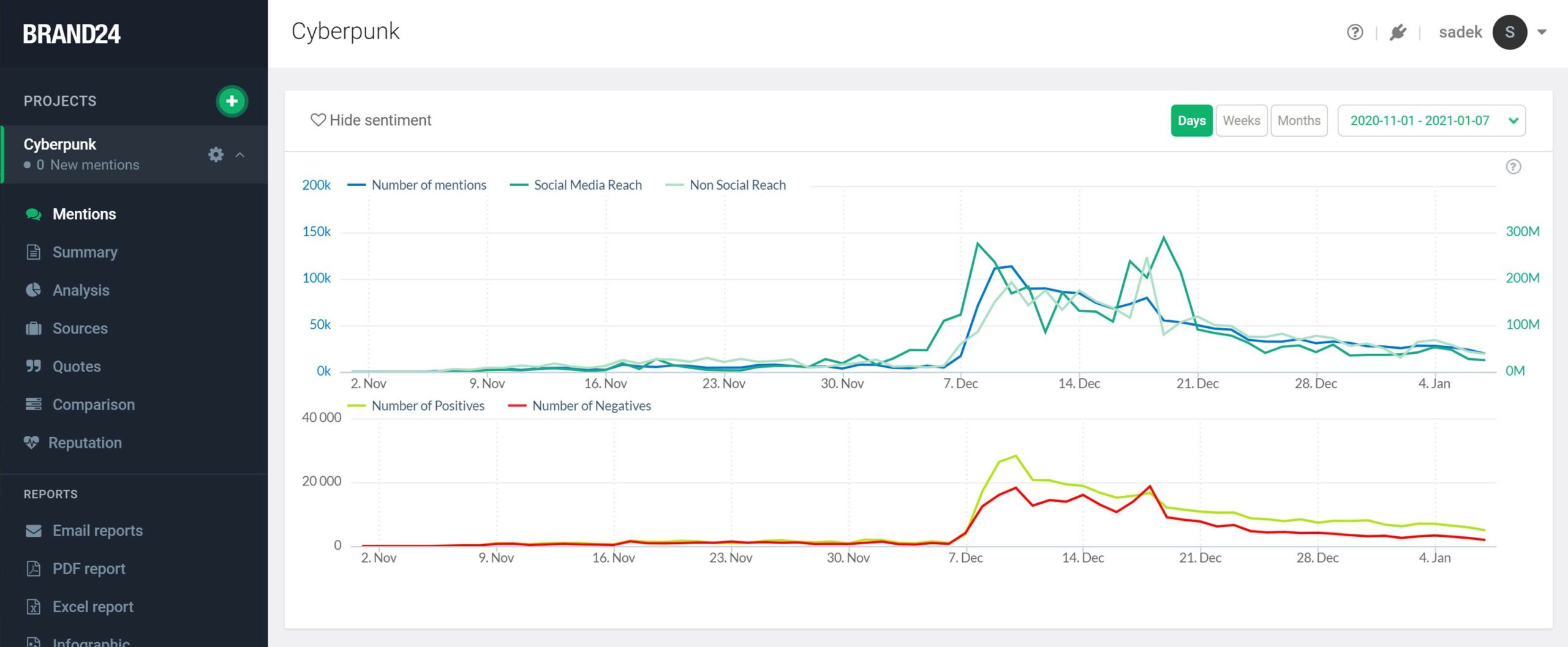
Sentiment analysis indicates that there was a significant predominance of positive sentiment over negative sentiment (quantitatively) during the period 7-14 December 2020. Would this indicate that such sentiment was confirmed by the upward trend in the price of CD Projekt shares listed on the WSE? Below we present the share price chart for this company (creator of Cyberpunk 2077) during the corresponding period.
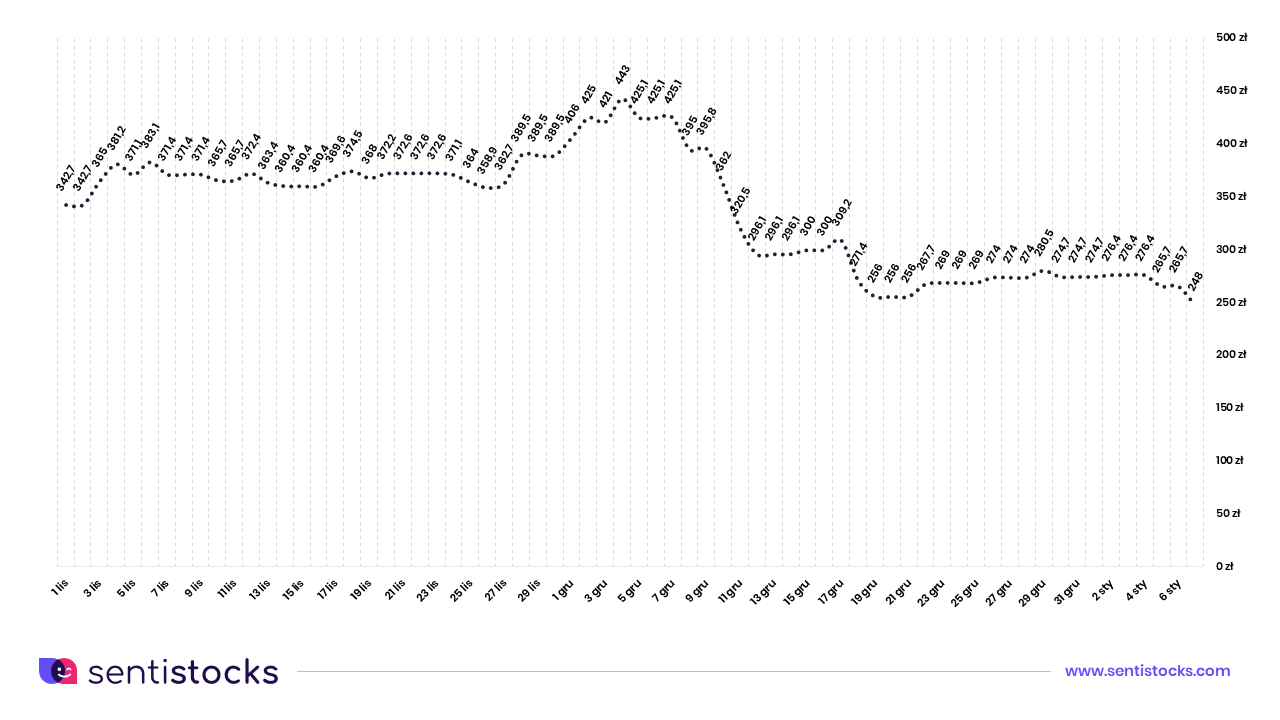
The share price chart shows the opposite situation from the sentiment chart. During the period in question (7-14 December 2020), CD Projekt’s share price recorded a significant decline, but the predominance of positive over negative sentiment visible in the tool did not indicate this at all.
Emotion analysis. Could you have predicted the stock market trend?
The answer is yes! Analyzing the intensity of the eight basic emotions (not sentiment) it was clear that two in particular stood out during this period. These were anger and trust. By observing the correlation between them we could see a specific trend behavior. We have additionally marked two key moments on the chart.
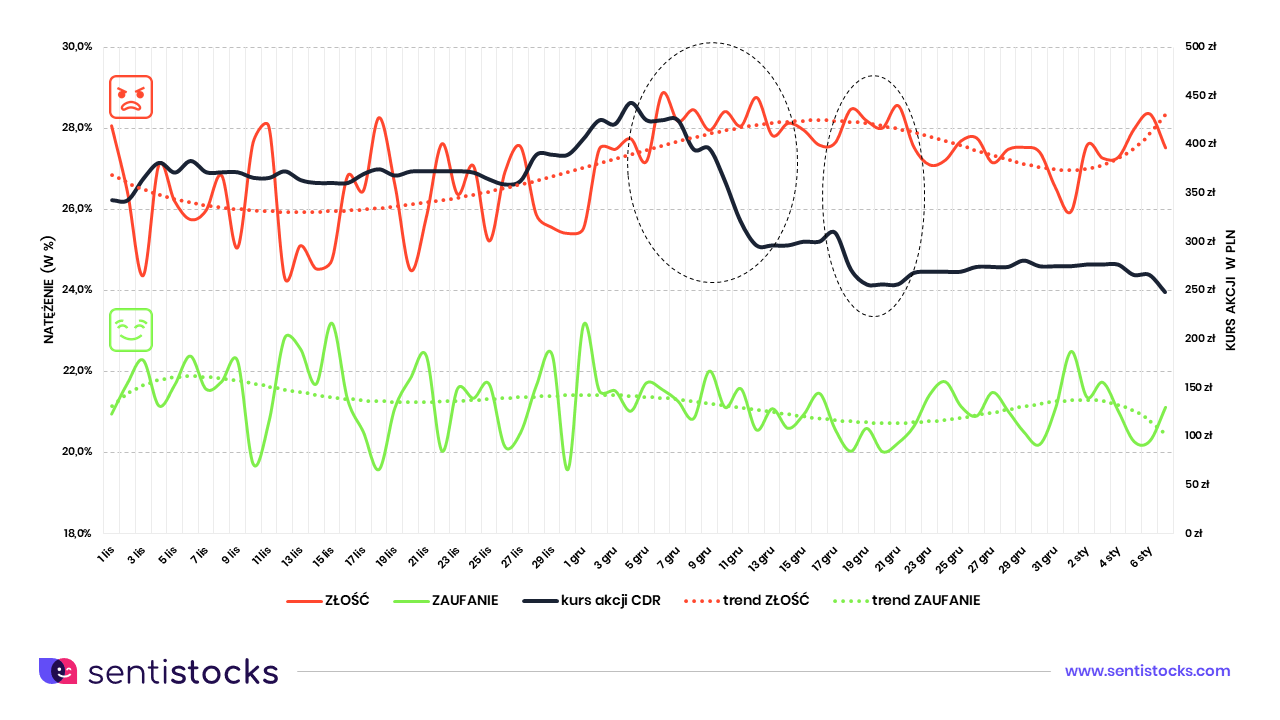
A 30% drop in the price of CD Projekt shares was recorded between December 7 and 12, 2020. Moreover, the period from December 17 to 19 last year saw another, this time 17%, price decline. It was during these time periods that the maximum divergence (i.e. the maximum difference in intensity) of the emotions ANGER and CONFIDENCE occurred.
The intensity of anger reached its maximum values with a simultaneous downward trend in the intensity of trust. What conclusion can be drawn from this? First of all the conclusion is that it was not the level of sentiment that signaled foreseeable drops of the company’s share prices. It was the emotions themselves, and more specifically the observation of changes in their intensity during a key moment for the company.
The analyzed case of the Cyberpunk 2077 game was a very simple case study for emotion analysis. It was accompanied by a huge information noise caused by the game’s release. The emotion analysis used in the SentiStocks tool therefore allows for very precise price predictions of various financial instruments. Therefore, its use can be a real support for investors in their decisions, and it can often help predict potential image crises.
At this occasion it is worth mentioning about even 85% effectiveness of emotion measurement in predicting the price of e.g. Bitcoin. Our tools perfectly cope with this. You can read more about this topic HERE.
by Sentimenti Team | Apr 7, 2020 | Sentistocks
Are emotions more effective on the stock market than the sentiment is? How effective are they? We have long been studying the importance of emotions in economic analysis. How they affect the prices of various financial instruments and values quoted there. We are not the only ones – for some time now, with increasing access to published opinions, whether on portals or in social media, there are publications and researches where authors try to find correlations between investors’ mood and stock, currency, crypto rates, etc.
December 2018 revealed the publication of “Media Sentiment and International Asset Prices”, issued by the National Bureau of Economic Research. In the aforementioned read, its authors have analyzed in Reuters’ and Bloomberg articles on their impact on major stock indices. Researchers discovered interesting relationships between sentiment and index values, amongst the others:
- The mood of the news correlates four times stronger with the bear market (dominated by declines) than during the bull market (boom),
- Emotions are a much better indicator of world indices’ behaviour than the commonly used VIX (CBOE Volatility Index),
- The positive mood in the media has a more positive impact on the developed markets, but a negative one on the emerging markets.
A similar topic was researched by Yigitcan Karabulut, Goethe University Frankfurt’s Assistant Professor. He analyzed predictions on a Facebook database.
None of the approaches, unfortunately, allowed the results’ accuracy of forecasts to be higher than 55-60%. This will not be possible whilst basing on sentiment or emotional tags offered by social networking platforms like Twitter or Facebook only. In Sentimenti, we have many more possibilities – up to 11 emotional variables. Do you want more information? Look below.
Sentimenti and the Warsaw Stock Exchange
In Sentimenti we took on another milestone in financial behavioral analysis. We use deep neural networks to analyze any published text based on 8 emotions and the overall arousal. Thanks to the idea we can read investor’s moods and predict their future actions more precisely. We have already proved it by checking our tools’ effectiveness on publications related to the Warsaw Stock Exchange. As many as 87.1% of the cases with shown changes in the emotion intensity area in texts about a certain company allowed us to predict the price changes.
Sentimenti and cryptocurrencies
For everyone who has even a little interest in the market, the cryptocurrencies are known for their strong emotional connection. Values such as Bitcoin, Ethereum (or others!) don’t have a real use so far but are mainly treated as purely speculative assets. And where speculation is concerned, there also are emotions.
That is why we have set ourselves the goal of checking how strong the correlation between cryptocurrencies and emotions is. And, by the way, does the same scheme work with the positive and negative sentiment only?
To that end:
- We have downloaded all available mentions of Bitcoin (BTC) for 2018 – over a million articles, comments, or posts.
- We have examined them emotionally for 11 indicators – 8 emotions, negative and positive sentiment, including arousal,
- for the tested period we have downloaded the price quotations, including opening, closing, minimum and maximum price, taking them hour after hour (over 35 thousand indicators).
We have then implemented our artificial intelligence to help. Our IT-team inserted all the data collected for analysis and set it the task for finding correlations between these several dozen variables, both on the side of stock market prices and emotions.
We have tested the 2018 datasets on a total of several hundred different training models. Then we started forecasting the best of them for 2019. We changed the approach several times, looking at the effectiveness each time. A few examples of this analysis ale pictured below:
The number of days analysed before the forecast day:
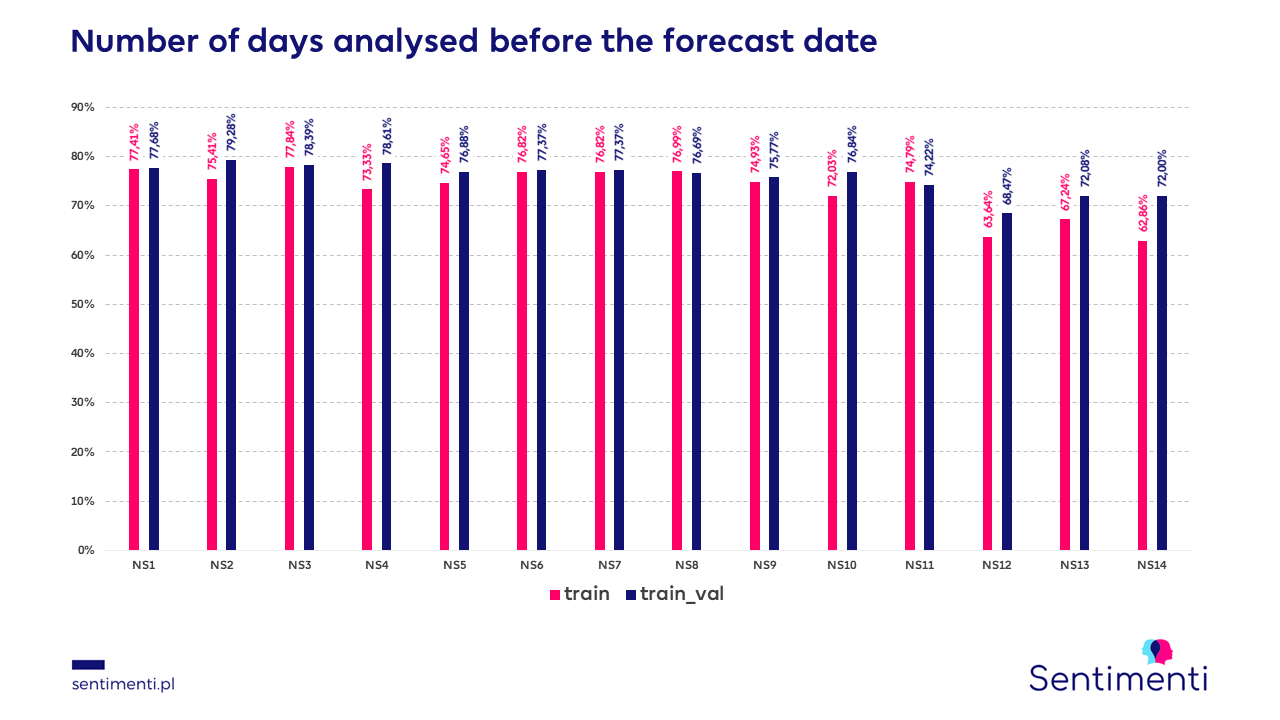
We checked which number of days back best affects the quality of the predictions. We examined periods from one to fourteen days. It turns out that the best results are obtained when we analyse the last three days (77.84% effectiveness of the prediction).
Removing one of the emotions
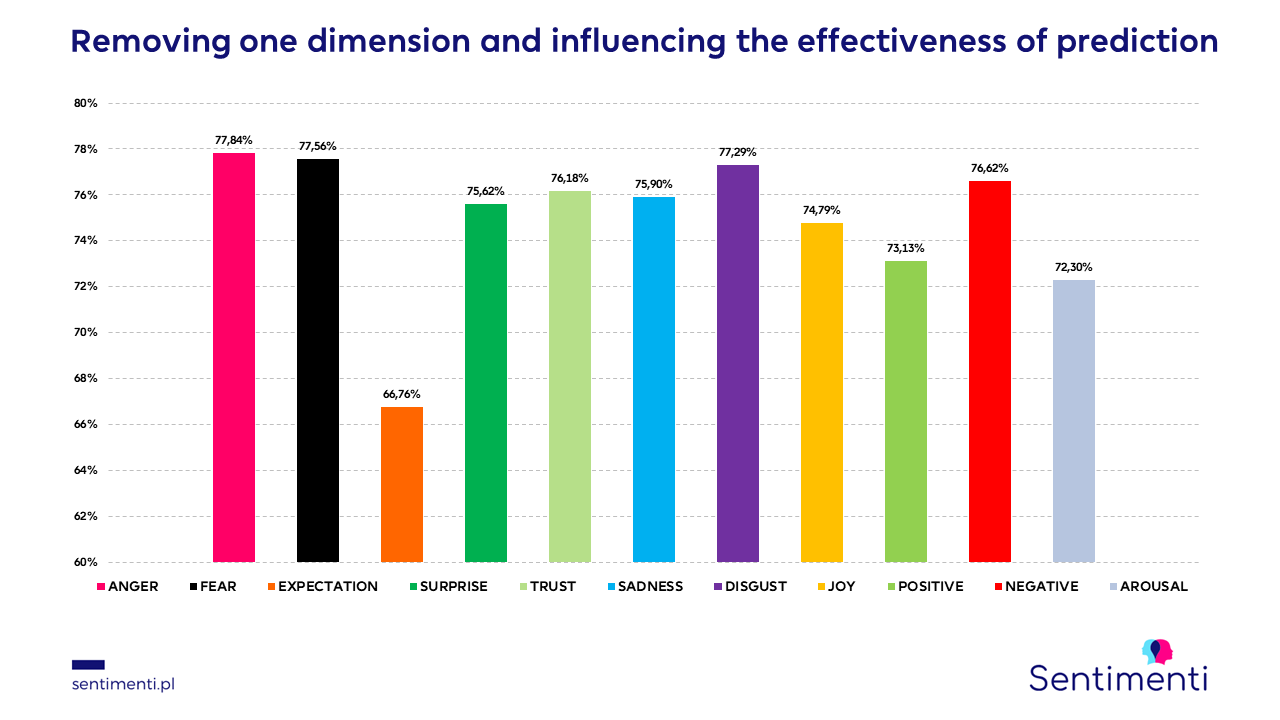
The number and choice of indicators considered are important. This can be seen in the graph shown above. Each of the variables influences the quality of prediction, it is enough to turn off e.g. emotion expectation for the quality to drop drastically. In other tests, we checked the effectiveness of prediction only on sentiment. Unfortunately, the results were far from the expected ones.
Quality of prediction vs. the time of the start of the 24-hour cycle
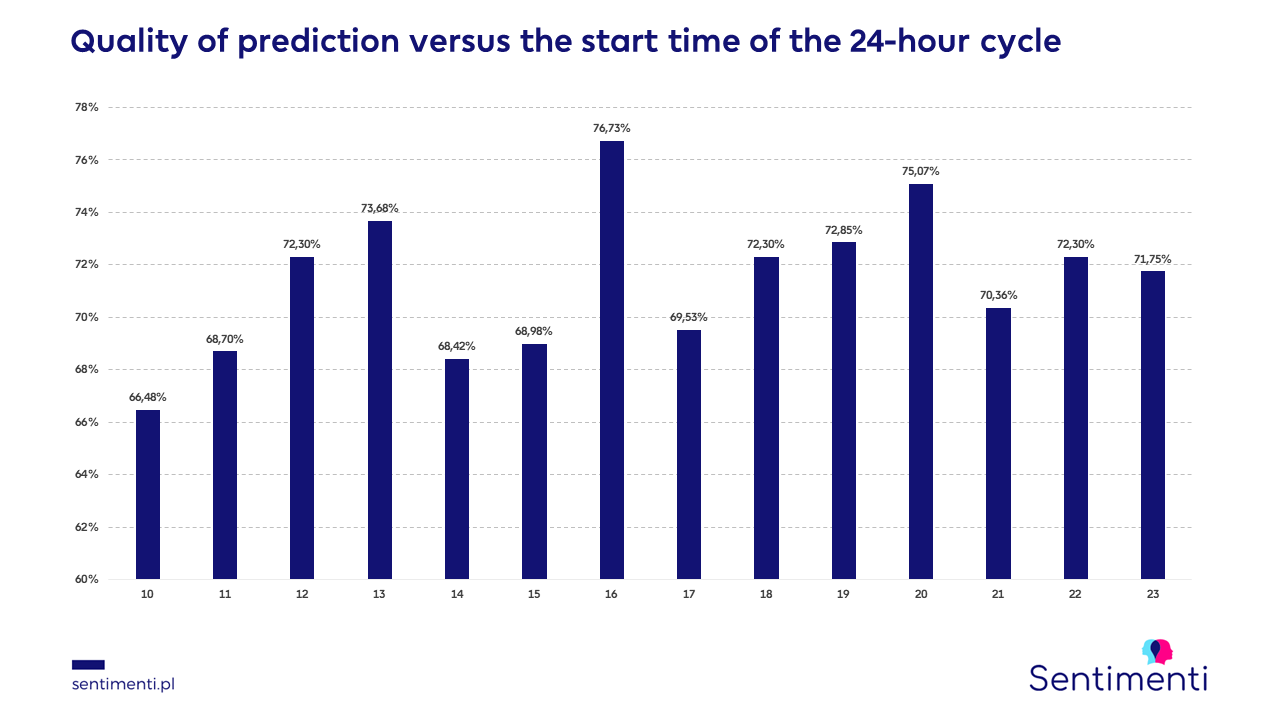
It turns out that the time when the forecasting starts is also important. In the time lapse between 10:00 and 23:00 the best results were achieved at 16:00 (76.73% effectiveness). This is the reason we take the hour to our daily forecasts.
Emotions on the crypto stock market: the final proof
After these and dozens of other tests, this is how the results of the best possible set of variables for 2019 (learned on the 2018 model) are presented:
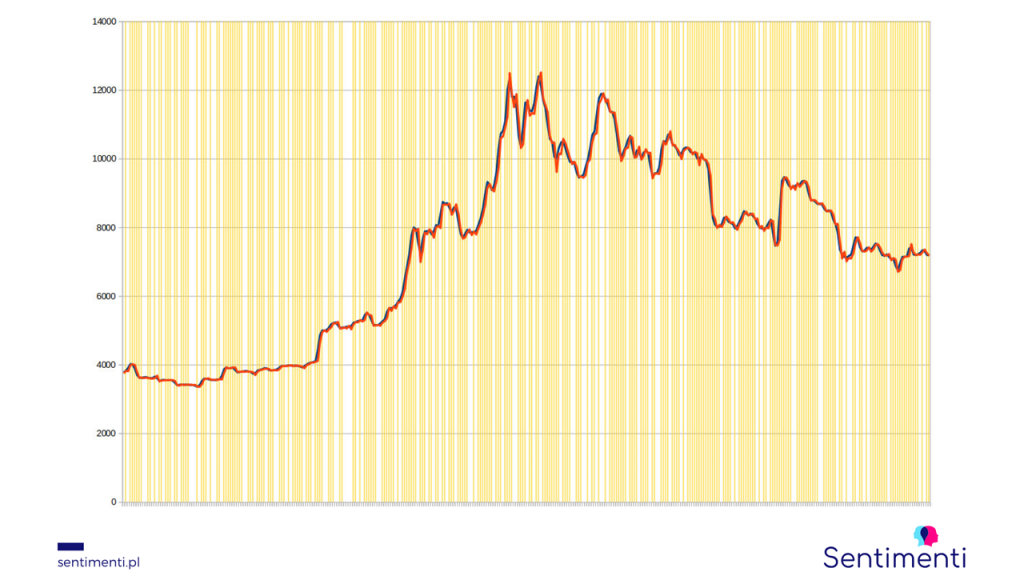
The X-axis is a period of 365 days. The Y-axis is the Bitcoin value in USD. Blue line is the real price, the red one is the forecast price. The yellow areas are the trend hits.
The prediction effectiveness, which is based on indicating the trend of price change, was 78.95%. As far as we know, such high effectiveness was not achieved in any of the previous studies researching emotions on the stock market. We are happy to see relatively small deviations between the actual and forecasted exchange rate (reaching a few percent at most). This is a good forecast for the already started tests of forecasting the numerical value of the course.
Take advantage of this knowledge and get access to information supporting your transactions. Measure emotions in the stock market and benefit from the results. We look forward to hearing from you!
by Sentimenti Team | Jan 27, 2020 | Lifestyle
The spring at Sentimenti promises to be an interesting one. When, in 2019, Sentimenti stopped being an NCBiR project and started as an independent business venture, we knew there were many challenges ahead. We created emotion analysis tools with very high accuracy, processing Polish based on the latest machine learning methods and linguistic knowledge. We are currently working with Interia or the National Cultural Center, and we want to develop further. Our plans include the English language, creating a plug-in for Chrome and improving the client panel.
Sentiments analysis – conquering English
Sentimenti’s tools “understand” the overtones of Polish-language texts better than most applications on the market today. We have long wanted to analyze other languages as well, primarily English. We will present the results of these plans as early as this spring.
The Sentimenti team is primarily linguists who created our tools and continue to improve them. Work on extending emotion analysis to English is already well advanced. They are coordinated by Dr. Jan Kocoń from the Wroclaw University of Technology, and he is supported by our chief developer Piotr Miłkowski.
A new artificial neural network is being developed, processing texts written in English in analogous ways to how our flagship product, emotion measurement for the Polish language, works. In our native language, we have achieved very high correctness, the errors of the automatic analysis compared to human responses do not exceed 2% of the intensity of any emotion, and the maximum deviation in a single text remains below 8%. We are confident that we will achieve comparable results in English.
The ability to analyze foreign-language texts will, as a first step, be used to develop the SentiStocks project, in which we analyze stock market trends and investor sentiment. Monitoring what is written about a given stock company and converting these mentions into a sentiment index has worked very well for the Stock Exchange. The addition of English will make it possible to expand the monitoring and thus make SentiStock available beyond the borders of our stock exchange.
Analyze any text and improve your
When we started working on Sentimenti, we focused on computational efficiency and the ability to process large volumes of data. This makes possible our collaboration with Interia, but also our daily practice of monitoring mentions on various, sometimes very popular topics and monitoring the brand against the industry.
However, we have always had in the back of our minds the voices of professionals involved in content marketing or public relations, who need tools to support them in creating texts.
We decided to create a plug-in similar to Language Tool or Grammarly. With it, it will be possible to check the tone of any text already published on a news site or social media. This functionality will be useful for all those who want to take a closer look at the language of the web. Our spring gift to writers will be the ability to also check your text and correct it on the fly. You will be able to see if the email you are writing expresses the emotions you had in mind. Does the promotional text really have a positive tone. Whether the press release is actually neutral.
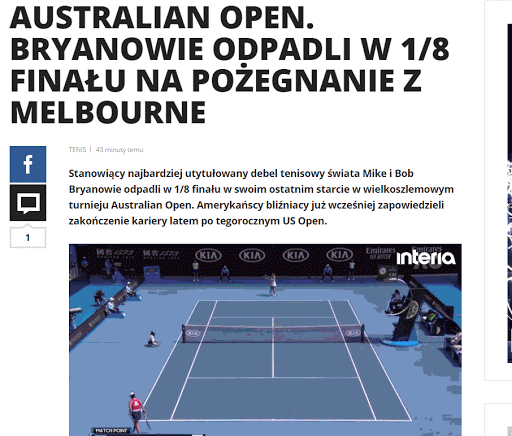
Plug-in for analysis of emotions in the text – the first fitting. If you would like to test this solution, please write to us, we will soon make it available to the public.
Spring at Sentimenti means new and nicer tools
Some of our clients are already testing the use of the Sentimenti panel. They have exactly the same tool in which our analysts work on a daily basis. It allows you to send XLSX and TXT files containing mentions or texts to the server for analysis and get the results of automatic measurement of emotions or classification of mentions.
The new Sentimenti panel will be much nicer and even more useful. In addition to handling communication with the server, it will also show us the initial results of emotion analysis – it will compile the averages of basic emotion and sentiment measures. It will also create ready-made charts that can be used in reports. In short, we are developing a dashboard for comprehensive emotion analysis.
Be with us in the spring
We are opening up to individual clients in need of text writing support tools. At the same time, we are sailing into the wide waters of the English language. We will be more user-friendly.
Sentimenti will probably forever remain a business-science project. We will not stop developing, we want to do research and improve our tools. Soon it will be possible to accompany us in several new ways.
by Agnieszka Czoska | Nov 8, 2019 | Conferences, Sentimenti research
Sentimenti = Emotions. We’ve previously discussed how to accurately analyze emotions with automated tools. Today, we’ll explain how we gathered the data that led to the creation of Sentimenti tools. This article is a guide to conducting effective research on the emotional meaning of texts.
The text was prepared for the GHOST Day machine learning conference and you can view the presentation in Polish.
Sentimenti. What emotions?
To train machine learning algorithms to automatically identify emotions in text, we first had to ask people how they feel. This seemingly simple question had to be broken down into several components.
First, what types of emotions should we consider? How many are there, and how do they differ? To answer these questions, we consulted the emotion specialists from the LOBI team. In psychology, there are various models of emotions, from simple to complex and multidimensional. We ultimately chose two models, which we now refer to as the sentiment and emotion models.
The sentiment model, based on Russell and Mehrabian’s 1977 paper, describes emotions along two axes: positive-negative and high-low arousal. As for the emotion model, we adopted the Plutchik model, both for its scientific robustness and because a portion of the Polish Slavic network had already been classified using it. This alignment allowed us to compare our findings with expert annotations, serving as a key test for accuracy.
What words?
Once we knew how to classify emotions, the next question was: what words to analyze? Our first step was focusing on the emotional meaning of words. We compared our findings with databases like WordNet and NAWL.
Our goal was to create a list of 30,000 words or meanings. Some words are ambiguous, with emotional tones shifting depending on context. For instance, “depression” can refer to both terrain and a mood disorder. We limited ourselves to a maximum of three meanings per word, each presented in context.
Thanks to the WordNet project, we learned that 27% of words have emotional meanings. These emotionally charged words took precedence in our analysis.
Sentimenti project: who participated?
To analyze emotional undertones in texts, we needed insights from a representative group of speakers. We worked with the nationwide research panel Ariadna to gather participants. Over 20,000 people took part in the study, providing data on at least 50 words each.
How We Collected Emotional Data
We designed a tool to assess word meanings on scales reflecting both sentiment and basic emotions. Participants evaluated words based on the emotional overtones they perceived in given phrases.
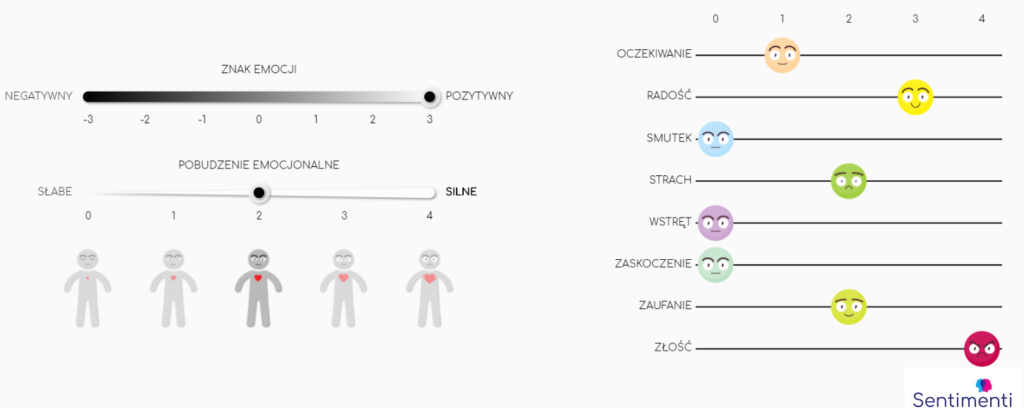
The study’s structure also considered participant fatigue. To ensure high-quality data, each person reviewed 150 words over three rounds, with breaks between rounds to maintain focus.
Beyond Words
Our next phase expanded beyond words to assess the emotional undertones of entire texts. Linguists have long known that the emotional meaning of a text goes beyond the sum of its words. The grammar and structure of a text also convey emotions.
For this phase, we analyzed existing reviews (e.g., hotels, doctors), as well as shorter forms like sentences and phrases. To ensure comparability with our word-level analysis, participants rated texts using the same emotional scales.
From People to AI
Sentimenti’s text classification tools now achieve high accuracy in identifying emotions, thanks to the solid dataset we built from word and text evaluations. While advanced neural networks may seem impressive, no AI can succeed without robust data to train on.
We’ve shared the details of our algorithm development both on our blog and in this scientific publication. Additionally, 20% of our word database will soon be published for researchers worldwide who study Polish emotions. This interactive database will have its own dedicated page, similar to NAWL’s list of affective words.
by Sentimenti Team | Jul 10, 2019 | Conferences, Scientific publications
Place of publication:
Proceedings of the 10th Global Wordnet Conference
Title:
Propagation of emotions, arousal and polarity in WordNet using Heterogeneous Structured Synset Embeddings
Authors:
Jan Kocoń, Arkadiusz Janz
Abstract:
In this paper we present a novel method for emotive propagation in a wordnet based on a large emotive seed. We introduce a sense-level emotive lexicon annotated with polarity, arousal and emotions. The data were annotated as a part of a large study involving over 20,000 participants. A total of 30,000 lexical units in Polish WordNet were described with metadata, each unit received about 50 annotations concerning polarity, arousal and 8 basic emotions, marked on a multilevel scale. We present a preliminary approach to propagating emotive metadata to unlabeled lexical units based on the distribution of manual annotations using logistic regression and description of mixed synset embeddings based on our Heterogeneous Structured Synset Embeddings.
Link: ACL Anthology
Citation BibTeX:
@inproceedings{kocon-janz-2019-propagation,
title = “Propagation of emotions, arousal and polarity in {W}ord{N}et using Heterogeneous Structured Synset Embeddings”,
author = “Koco{\’n}, Jan and
Janz, Arkadiusz”,
booktitle = “Proceedings of the 10th Global Wordnet Conference”,
month = jul,
year = “2019”,
address = “Wroclaw, Poland”,
publisher = “Global Wordnet Association”,
url = “https://www.aclweb.org/anthology/2019.gwc-1.43”,
pages = “336–341”,
abstract = “In this paper we present a novel method for emotive propagation in a wordnet based on a large emotive seed. We introduce a sense-level emotive lexicon annotated with polarity, arousal and emotions. The data were annotated as a part of a large study involving over 20,000 participants. A total of 30,000 lexical units in Polish WordNet were described with metadata, each unit received about 50 annotations concerning polarity, arousal and 8 basic emotions, marked on a multilevel scale. We present a preliminary approach to propagating emotive metadata to unlabeled lexical units based on the distribution of manual annotations using logistic regression and description of mixed synset embeddings based on our Heterogeneous Structured Synset Embeddings.”,
}